A simple tool for qualitative research and inquiry, also useful for planning and evaluation
Contents of this page
-
- Background
- What is Hierarchical Card Sorting (HCS)?
- What use is it?
- How do you do it?
- Examples of HCS results
- Two user accounts of the use the HCS
- Problems and limitations
- Making use of HCS results
- Aggregation of multiple HCS exercise results
- Future directions
- Earlier methods
- Extra references
- A pdf copy of this page
Background
This is a tool I developed in 1993, while doing PhD field work with the Christian Development Commission of Bangladesh (CCDB), in Dhaka, Bangladesh. I subsequently wrote two how-to-do-it papers describing the method (both of which have been on the web for some time):
This page now integrates the contents of those two papers, and adds further content developed since then. An initial clarification may help. Hierarchical Card Sorting refers to the process. Treemaps refer to the product of that process.
What is Hierarchical Card Sorting (HCS)?
HCS is one of many types of card sorting methods (also known as pile sorting). Card sorting has been used in many contexts, from traditional ethnography to the modern day business of designing usable websites (See references below). In these contexts card sorting is typically used to elicit people’s mental models: (a) the categories they use, (b) what belongs to these categories, and (c) how the categories relate to each other.
What use is it?
In many organisations people accumulate a lot of knowledge, but often it is tacit and informal in nature. As such. it is not so easily shared. Yet sharing that knowledge can make a difference, other people can make use of it, and they can help correct it and improve it. A HCS can help make people’s knowledge more explicit and publicly available, contestable and usable.
How do you do it?
The HCS method asks people about significant differences. About differences which are important to them and which have (or had) consequences. It has similarities in origin and approach with the Most Significant Change (MSC) technique. Central to the HCS is a question about the “most significant [static] difference”, whereas MSC asks about the “most significant change”. Both ask respondents to make observations and interpretations. The design of both tools was influenced by Gregory Bateson, especially his book “Mind and Nature: A Necessary Unity” (1979), in which he argues that information is “a difference that makes a difference”. In turn, many people would argue that knowledge is structured information. The HCS is about eliciting and representing people’s knowledge (i.e as a structured set of differences that make a difference).
Normally the HCS is used with one respondent. However, the process outlined below can easily be used with a small group. The steps:
-
- Identify the respondent’s area of expertise or knowledge that you want to explore. For example, the knowledge of animal diseases held by a paravet, or knowledge of local NGOs held by a INGO staff member working on NGO capacity building. Examples of both below.
- Generate a list of actual cases which will be sorted. If possible, try to select cases that represent a wide variety of examples. In many cases you will want to select cases that the respondent is responsible for in some way, and thus should be expected to know about them. For example, a list of cases treated by the paravet in the last month, or a list of NGO grantees managed by an INGO desk officer. Don’t be too ambitious, especially to start with. Large numbers of cases (25+) will make the process more time consuming and will run the risk of boring the respondent and interviewer. Write the name of each case down on a separate card. Cases might be events (treatment provided) or entities (clients).
- Place all the cards in one pile (see this as the trunk of a tree) and begin by asking the respondent to tell you about some of the differences between all these cases. The purpose of this initial question is simply to generate a shared awareness of the large number of differences that (inevitably) exist. It is a warm up exercise. If the respondent finds this difficult, randomly select two cards at a time, and ask them to compare them, with a view to finding interesting differences.
- Ask the respondent to sort all the cards into two piles of any size (see these as the first two branches above the trunk of a tree), representing what they think is the most significant difference between all the cases represented on the cards. Emphasise that it is their opinion of “significant” which is important. If you want to direct their attention in a particular direction then use a prefix to the question, such as “In your roles as…what do you think is the most significant difference between…?. Or “Considering the objectives of this organisation…what do you think is the most significant difference between…?
- Emphasise that a distinction is significant if it makes a difference. Because respondents may casually offer a difference simply to oblige the interviewer it is important to check its significance by asking “What difference does this difference make ?” If one can’t be identified then suggest to the respondent that they consider if there are other differences which might be more significant.
- Keep a record of which cards are placed in which pile. This is easier if all the cards have all been numbered beforehand. And then write down a description of the reported difference between the two piles. And what difference that difference makes.
- Take one of the two piles at at time and repeat stages 4 to 6 above. Then repeat this process with second pile. There should now be four piles. Repeat the same process with these piles until there is only one card left in each pile. One way of keeping track of this process is to use a large piece of paper, to draw a tree whose branches split into smaller and smaller branches.
- You can develop the tree structure in various ways: (a) breadth-first, or (b) or depth first, or (c) a mix of both. Your choice might best be guided by where the respondent seems to be most at ease.
- In some cases there may be more than one example left in a pile but the respondent may not be able to identify an important difference between them. Don’t force them to do so, but simply note that no further difference could be identified.
- Document the results of the exercise in a form that shows how the different groups and sub-groups or cards are nested, along with their perceived differences and the differences these make. See the next section here for examples.
- Other steps can then be taken to make use of the knowledge structure elicited by the HCS. See Making use of HCS results below.
Examples of HCS results
Individuals as respondents
The HCS process generated tree structures, which can be represented in a table or diagram form. Figure 1 is a tree in table form (using Excel), produced in 1993. Click on the image to read the text, and click again to enlarge. Read the results from left to right. Green shaded sections each refer to one of two binary types that the respondent would like to see funded more in the future – information that was solicited after the tree structure was developed (more on this below). These preferences have then been converted to an overall ranking, as seen on the right. This example is incomplete because while it shows differences, it does not yet show the differences they make i.e. the expected or realised consequences.

Another example is shown in Figure 3 below. This is the result of an interview with a rural community development worker, where I asked about the most significant differences between the villages they were working with. The wealth ranking on the right is based on binary comparisons made at each junction of the tree, by the respondent. After the tree had been constructed, I had asked them which of the two sub-groups of villages at each junction they thought were more versus less poor.


Groups as respondents
Figures 5 and 5b were developed by two small groups, rather than by one single respondent. This exercise was one activity within larger planning process, designing a new rural livelihoods programme in Nigeria in the late 1990s.

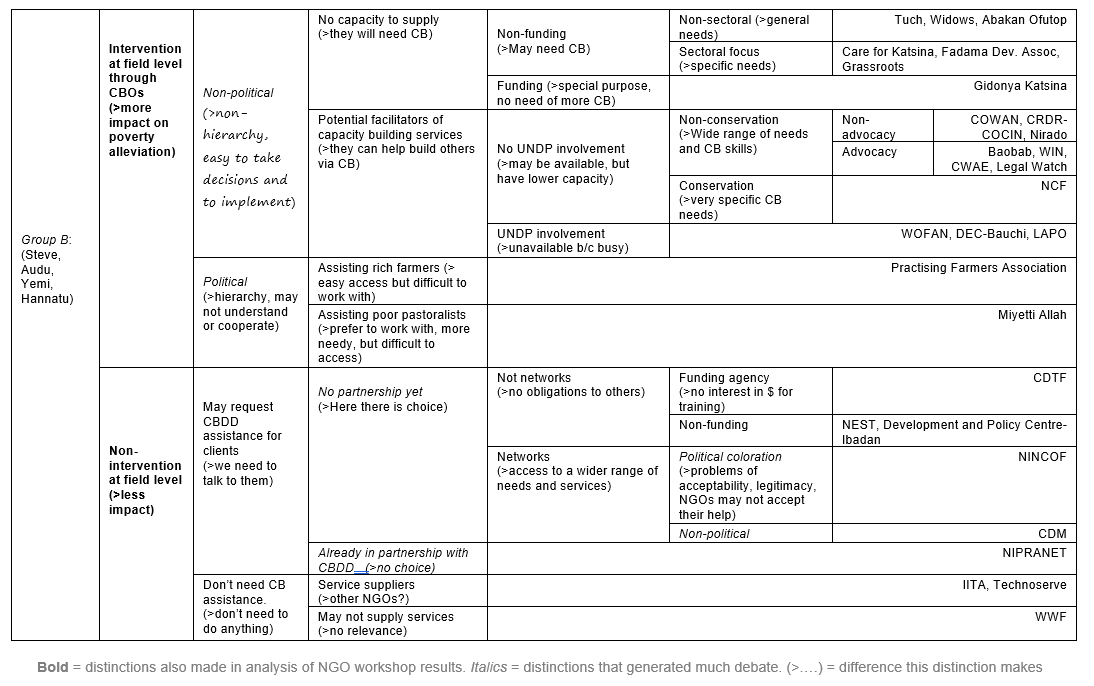
HCS with urban communities in Iraq in 2021
This 2021 USAID report describes the work by Niki Wood, Sarah Ghattass, and their Chemonics colleagues with a USAID project in Iraq. The respondents in the HCS exercises there were Community Coordination Officers, who were each working in different urban locations in Iraq, with the common aim of increasing community cohesion, capacity for conflict resolution and economic development. The entities sorted in each HCS exercise were various identifiable social, cultural, ethnic and demographically defined groups present in each of those locations. There is also a blog posting here, about that experience
Two user accounts of the use the HCS
Bangladesh
In 1994 staff of the Research Unit of the Christian Commission for Development in Bangladesh (CCDB) used the cardless version of the same method in the process of exploring poor peoples conceptions of health, disease and medicine. The following quote describes the results of two applications of the method:
“In two somities [beneficiary groups] we also asked the members to come up with different medicaments they use when they are ill. We asked them to divide these medicaments into two groups. We asked for an explanation after each division. After dividing the group into two other groups we asked them to do the same for the two other sub-groups. In the first somity they divided the medicaments into allopathic and herbal medicines. The allopathic medicines were divided into tablets and syrup. The herbal medicine into medicines used for cough and influenza, and medicines for influenza.
In the second somity they divided the medicaments into medicines given by the kobiraj and medicines given by the allopathic doctor. The medicines given by the kobiraj were divided into medicines for weakness and medicines for influenza and headache. The medicines of the allopathic doctor were divided into medicines for pain and medicines for gastric burns.
We used this exercise to get more information about people’s concepts of medicines. When we asked about [the consequences of] the difference between herbal and allopathic medicines it was mentioned that allopathic medicines cure better and sooner but they are costly and can give weakness. Herbs are “softer” to the body, they don’t harm the body, they are cheap , and although they work slowly they keep the body healthy.” (People’s Health and Nutrition, January 1995, CCDB)
Afghanistan
“I thought I would let you know that I have tried your treemap method for the first time. Despite thinking it sounded rather unlikely to be useful when I first read it, it has provided a very nice alternative to classic wealth ranking for investigating the structure of villages. The people I am working with had the same unease as I do about launching into wealth analysis. We therefore tried your method as it seemed to offer a way round the problem. We ask the number of families in the village, then ask about differences between them with respect to livestock and well-being. Following the process as you describe, we rapidly get a good idea of the wealth structure of villages, which is far more disparate than I had imagined. From this exercise it is possible to find people to interview in more detail from each category. Repeating the exercise with paravets, we then asked them to indicate what proportion of their work was with which group. This worked well and provided an indication of who was benefiting most from the services of the programme.”
Problems and limitations
1. Some caution may be appropriate. Like all participatory methods it requires some trust and confidence in the relationship between yourself and the person whose views you are seeking. Secondly, there is no guarantee that the views that are expressed will be stable over time. Peoples views of the world change, and the expression of their views is often very context dependent.
2. Some people react at some stage to the exercise by saying “There is no difference between these“. Here I have cautiously tried to give many examples of possible differences, while being careful not to lead in any particular direction. I have emphasised that differences can be found even between objects that look identical, the question is which of these is most important from their point of view. I also emphasise that we are looking for relative rather than absolute significance. But if the person is really struggling, especially after having already identified previous differences between the earlier bigger piles, I do not push them.
3. Another problem is almost the opposite in nature. People can approach the task in what appears to be an un-engaged manner, blithely tossing off distinctions which don’t seem too significant. When this happens I have tried asking “In what way is that significant, what difference does that make ?, checking to see that the respondent can articulate the significance, and if not checking to see if they really understand the exercise.
4. Another problem relates to respondents who are almost too helpful. As can be seen from the tables below it is common for some respondents to report more than one difference. When well organised I have dutifully noted these down and then asked the respondent, after reading them back, “and which of these……are the most significant ?” Failing to do this has meant I have been the one that ends up speculating on their relative importance to the respondent.
5. Many respondents find it easier to identify differences, than it is to identify the differences these differences will make. Often both respondent and interviewer assume that this is self-evident and fail to document this part of the exercise. Yet these beliefs can contain important assumptions or hypotheses about the way things work, which can benefit by being openly described, scrutinised and tested.
Making use of HCS results
Value can be obtained from tree diagrams at two stages:(a) during the creation of the tree diagrams, and (b) through comparisons made between parts of the structure once it has been created.
Ethnographic use
During creation of a tree diagrams the main use is as a ethnographic tool: a means of understanding people’s view of the world. There are three types of use:
-
- Identifying the distinctions that people see as relatively important. This is evident in the contents of the differences reported. It is also evident in how early in the exercise they are reported, and how often they are reported (on different branches).
- Identifying the limits to people’s knowledge: When respondents cannot identify differences between two or more entities the limits to their knowledge seem to have been reached. Knowing what people do not know about can be important, especially when they might be expected to, or claim to have, expertise in that area.
- Identifying the direction of learning: It is also worth noting where there is more versus less differentiation of knowledge, visible respectively where where branches end up with a single case rather than multiple cases, which have not yet been differentiated. See Figure 1 above for an example.
Planning and evaluation use
Exploring
After creation, the tree structure can be used to ask a series of comparison questions. At each junction respondents can be asked questions about the available binary choices i.e. between the two alternative branches. The default process for soliciting and documenting these judgments is to proceed from the trunk to the leaves. At least four different kinds of questions can be asked:
Questions about: | In the past | In the future |
Differences in degree (more vs less) | 1 | 2 |
Differences in kind (categorical) | 3 | 4 |
Some examples of each of these types of questions are:
-
- “Which of these two groups do you think has been most successful, so far?” [1]
- “What is the most significant difference in the nature of the achievements of these two groups, so far” [3]
- “Which of these two groups do you think will be most successful over the next x period of time?“[2]
- “In the next six months, how will your work with this group be different, compared to this group?” [4]
- “Which of these two groups do you expect will present the most problems in the next six months?” [2]
- “What do you think will be the most significant difference in the problems face by these two groups, in the next six months?” [4]
- “Which of these two groups do you plan to be spending more time with in the next six months ?” [2]
The answers, and associated rationales, can be added as a further annotation to the tree diagram, at the relevant junction.
Caveat: Sometimes people may not be able to make a make a choice (of degree) or provide a choice (of kind). In which case, simply note this fact, and proceed down the tree structure to the next binary distinction.
Ranking: In addition, the answers to all of the binary “more versus less” questions can be used to generate an overall ranking of the items in the set that has been sorted. See Figures 1 and 3 for examples. This can be done by examining each branching point, and re-sorting – where necessary – the two sub groups such that the one identified as “more …” is at the top, and the one identified as “less…” is at the bottom. When this is done with all branching points, then the aggregate result will be a completely ranked set of entities, still with the basic network structure of the HCS tree intact.
The ranking that is produced can be seen as a kind of inductively generated “rubric” i.e. an annotated scale. As well as capturing the full diversity of cases that are sorted, this scale provides an opportunity for identifying a transparently assessable “cut-off” point, above which performance or eligibility is regards as being acceptable.
Testing
Reiteration
A HCS can be carried out at multiple points in time, prior to, during and after an intervention. In order to: (a) identify current status of the people/organisations being compared, (b) to compare these with past assessments, and (c) to detail plans for the upcoming future. This kind of application would be most useful in situations where there was a diversity of contexts and needs, and the need for variations in the specifications of interventions.
Scatter plots
Once you have two or more sets of ranking for the items that have been sorted the relationship between these can be visualised in a scatter plot. Here is a good argument for more use of such simple devices
Creating a testable predictive model
Each branch of a tree diagram can potentially be seen as a causal configuration, i.e.a set of conditions associated with an outcome seen in the entities that have been sorted . A complete set of branches can then be seen as a particular kind of Theory of Change, one that is notably different in at least two ways.
-
- Firstly, because there will be multiple branches – it will be capable to representing equifinality i.e the reality that there are often multiple alternate means of reaching the same outcome. It will also be capable of representing asymmetrical causal processes i.e. where an outcome can be absent not because of the absence of its usual causes, but become of the presence of other influences.
- Secondly, in contrast to more conventional representations, each segment in a branch is not a consecutive event, forming a sequence of events. Rather, each segment is an additional kind of difference that is expected to make a difference to the outcome observable in the entity at the end of each branch.
PS: This type of tree structure can be seen as a type of “Ethnographic Decision Tree”, a variant of a method developed by Christina Gladwin, in the 1980s.
As can be seen in Figures 1 and 3 above (and explained here) it is possible to convert a set of binary predictions about expected relative success into a ranking. This ranking data can then be compared to independent measures of success, also converted to rank value, to test the validity of the elicited predictive model. Two sorts of comparisons can be made:
-
- Using variable values: Calculate the correlation between the two sets of rank values, using Spearman Correlation (see how to do that in Excel)
- Using binary values: Convert the ranking values into binary categories (1>middle rank value, 0=less). Then summarise the distribution of these value in each of the four cells of a a Confusion Matrix. In the table 6 example below the 27 items were projects within one of a grantee’s portfolios. The card sort described significant difference between the projects and the measure of project success was obtained from a sperate monitoring system.
In this analysis each set of ranking values has been dichotomised into higher versus lower rank values (above/below the median rank value). The distribution of values in the table can then be evaluated using range of different measures. One common measure is Accuracy (= True Positives + True Negatives/All cases). In this instance the HCS predictions had an overall Accuracy of 59%

Experiments with different cut-off points can often identify a better performing prediction model. In this example, lowering the cut-off point for the predicted success status to between 7 and 8 (the lowest ranking available) increased the performance to 74%.
The False Negative (FN) cases are where one or more other configurations (within the “less successful” ranked cases) might be responsible for the “more successful” status. The False Positive (FP) cases are where other features outside the configurations (within the “more successful” ranked cases) must have some yet-to-be-identified role, and be responsible for their “less successful” status.
Although the binary approach (aka classification vs regression) seems to involve loss of data, in this instance it looks like it may have been more informative. The correlation coefficient for the ranked values was only 0.135, which suggests on first glance that there was no relationship here worth looking at.
Other Applications
- Assessing capacity building activities: When supporting capacity building work with individuals or whole organisations, we might expect that this assistance, either in the short or long term, would make a difference to the assisting person or organisations relationships with their clients. For example, the service provider might be more sensitive to the differences between client’s needs. Or, they may also be more up to date in their knowledge about their various clients’ needs. Or, the differences they see between their clients (that they think are significant) may be more reflective of their clients concerns, and not just their own. That knowledge can be elicited during a HCS process, where the clients names are the items being sorted.
- Doing a stakeholder analysis. It is possible to use tree diagram as a means of doing a stakeholder analysis in a development project. This can initially be from the perspective from one observer, possibly an individual stakeholder, such as project manager. Firstly, a list of actors reflecting the maximum possible variety of stakeholders are identified. The process of inquiry then starts at the trunk, with the respondent being asked to identify “the most significant difference between all the stakeholders in the project”. Optionally, after a prefix saying “Bearing in mind the objectives of this project…” . This is then followed by a question about the difference that difference makes i.e. the actual or expected consequences. Then each of the two initial categories of stakeholder are progressively differentiated until all cases are located as a leaf of their own.The net result is a nested classification of all the listed stakeholders. Information is generated not only about the differences between types of stakeholders, but also about the consequences, past, present or future of those differences.
- Portfolio analysis: “Most measurement and adaptive management approaches were developed for and from individual projects (Sweetman and Conboy, 2018). Senior managers and public officials, however, are often interested in results at more aggregate levels, looking across multiple projects at wider portfolios of work” (Buffardi et al, 2019). Because a HCS is focused on a collection of entities, rather than one entity, it has potential as a useful tool for portfolio type analyses. The following purposes and questions associated with portfolio analyses, identified in the above ODI paper, could be addressed by a HCS exercise:
- Overview/health check: What areas need more attention?
- Hypothesis testing: Which approach works better? What should be scaled up, down or discontinued?
- Balancing/hedging: How can the portfolio maintain a pipeline of outcomes over different time frames, which range in their likelihood they will be achieved and level of risk
- Comparative advantage/future positioning: In the next five years, how can we maximise the value of our investment and unique contribution relative to others? What should we move out of and expand into? How should future resources be allocated.
- Intersectionality: “…is an analytical framework for understanding how aspects of a person’s social and political identities combine to create different modes of discrimination and privilege“… An intersectional analysis considers all the factors that apply to an individual in combination, rather than considering each factor in isolation” (Wikipedia) HCS could be easily used for these purposes. Firstly, by re-iteratively eliciting respondent’s views of the “most significant difference” between different people (groups or individuals), such each has their own composite identity, but which is shared with others when viewed at different levels of aggregation. Secondly, by then posting exploratory binary choice questions about past or proposed differences in interventions affecting different groups and sub-groups.
- Analysis of Most Significant Change (MSC) stories: MSC stories can be treated as items to be sorted, using the HCS process. The resulting tree structure will provide an overview of all the MSC stories, at multiple levels of detail. Binary exploration questions can then be asked, that make use of the same tree structure. Asking about, for example, how the respondent thinks an organisation should respond differently to each type of change described in the MSC stories
Aggregation of multiple HCS results
If multiple individuals are asked the the same HCS question then their results can be aggregated, and then analysed, with at least two different purposes in mind:
-
- Identification of similarities and differences between different people’s views of the world. This can be done using qualitative and quantitative methods, as described below
- Identification of a synthesised model, one that best predicts an outcome of interest, based on the views of all the participants in the HCS exercises
Qualitative methods of aggregation
The first method is qualitative, and involves what could be called a macro-HCS. Each HCS sort result is in effect treated as a new type of “card” or case. Participants are then asked to identify the differences between these different HCS results. Firstly to generate a list of such differences, in order to reflect on them. Then to select “the most significant”, on a reiterated basis, generating a tree diagram, of the kind already seen above. Figure 8 is an example of a Macro-HCS, using each of the previously described HCS results as “cards”

A variant of this approach could be called a meta-HCS. In Figure 8 the “cards” (i.e. HCS exercises) have been differentiated on the basis of the different types of cases they each analyse (i.e. NGOs and villages). But if all the HCS exercises were using the same set of cards (e.g., same set of NGOs), then the Figure 8 Meta-HCS would have to focus on differences between the kinds of differences documented in each HCS.
I think the following Figure 8b example qualifies as a meta-HCS. In 1992 I interviewed the chief executives of 32 largest NGOs in Bangladesh. In that interview they were asked “What is the most significant difference between this and other NGOs [in Bangladesh]?”. I summarised their answers by asking myself the same question, but with the CEO’s distinctions between the NGOs being the objects of concern. Figure 8b is the HCS tree tree structure that I developed using the reiteration of that question as built into the HCS method.

For reflections on the significance of the contents of this structure see pages 161-163 of my 1998 PhD thesis on organisational learning in NGOs
Quantitative methods of aggregation
Aggregation, using social network analysis software
This can be done with two different types of card sorting results: (a) results of multiple HCSs, or (b) results of multiple “free sorts”, where each respondent is asked to sort cards into 2 or more piles, on a once off basis (i.e. not reiterated)
Aggregating multiple free sort results
This is a relatively simple process. Cases (sorted items) are listed row by row. Successive columns describe the attributes of multiple pile sorts. The first two columns describe the names and members of the first respondents twp piles. Or the first three columns, if they sorted into three piles rather than two piles. The next set of columns then describe the names and members of the second respondents pile sort, etc.
Remember that pile sorts can be used to describe either: (a) differences between entities in terms of their outcomes to date. Or, (b) differences between entities in attributes like context and activities, which were expected/thought to make a difference to one of those outcomes of interest.
Aggregating multiple HCS results
Step 1: The results of each card sorting exercise need to be converted into what is called an adjacency matrix format. The cells in the matrix show if a row card was placed in the same pile as a column card and if so how many times that happened (0-N). An example is shown in Figure 9 below. This was generated using the results of the Figure 5a card sorting exercise shown above. Bear in mind that because this is a HCS, any card is likely to be in multiple categories. In a free sort they are likely to be in one or the other category only.
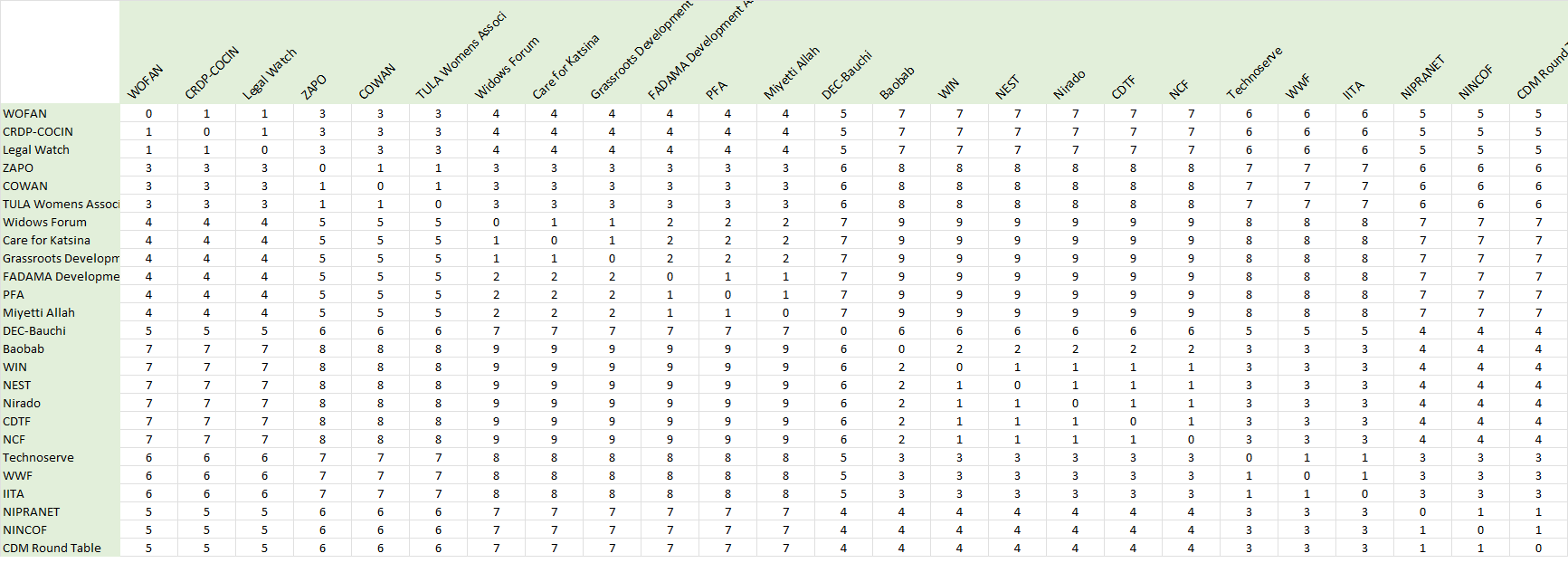
Step 2: The same process is repeated for each additional hierarchical card sort that has used the same list of entities to be sorted. The cell contents of each of the adjacency matrices are then added up, either manually or using Ucinet>Transform>Matrix Operations>Between datasets>Statistical Summaries. The summary matrix that is then generated can be used as an input to a hierarchical cluster analysis using Ucinet> Tools>Cluster Analysis>Hierarchical>Dissimilarities&Tree diagram. This will generate tree structure like Figure 10 (Caveat: This one only represents figure 9 matrix, not multiple aggregated matrices of that kind). Cards joined early on the left are most similar in their category membership, compared to those joined further to the right
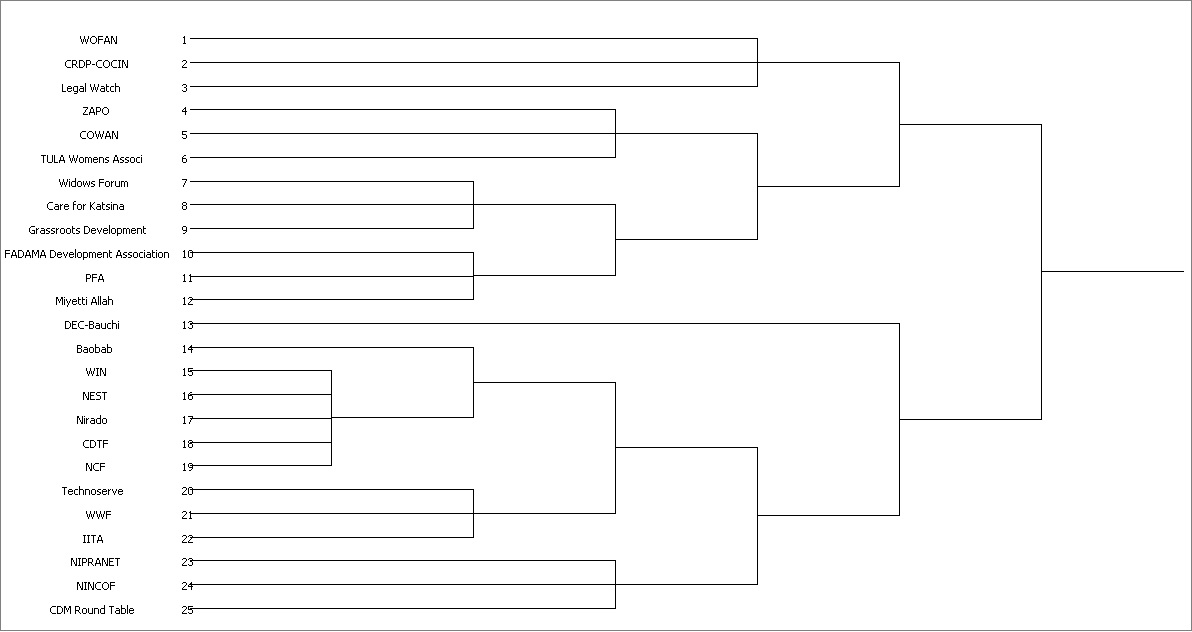
Step 2: Unlike the examples shown earlier above there will be no text invitations explaining what each of the branching point involve. These descriptions will need to be generated by the analyst by comparing the sets of entities that meted each branching point and identify what they think is the most significant difference between these sets.
Incidentally:
-
- For those interested in other ways of aggregating tree structures, a lot of work has been done in this area in the field of phylogenetics
Synthesising, using prediction modelling
Another option is to use a specific kind of supervised machine learning algorithms known as decision trees, to search and find combinations of attributes (from among all those identified by all participants), which are good predictors of the presence or absence of the outcome of interest. This can be done using Rapid Miner Studio, or EvalC3.
The simplest way of doing this is to generate an affiliation matrix, using results of multiple participants free sorts. Here rows = participants, columns = pile names, cells = membership of pile (0/1). In addition, an extra column needs to be added which describes the status of the outcome of interest, as an extra column on the right side of the data set.
With HCS results it is more difficult, because they require more follow up work.. The same kind of affiliation matrix will have many gaps, where cells have no values See figure 11 (based on figure 5a). Here follow up work interviews with respondents may be needed. Missing values will arise because many bifurcations in a HCS tree structure only involve a sub-set of all the cases.

After either of the two kinds of the data set has been prepared Rapid Miner or EvalC3 can be used to generate a decision tree, also known perhaps more appropriately as a “classifier”. Here is what a Rapid Miner decision tree looks like (using variable and binary data):
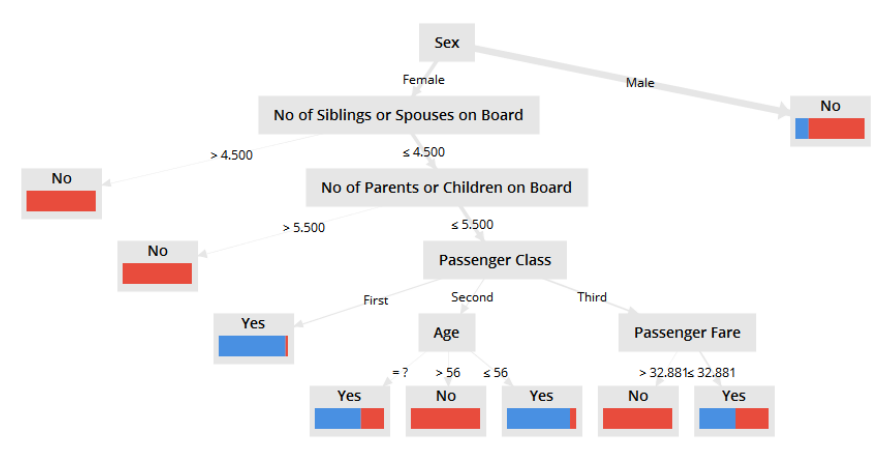
Future directions
Software
A web-base software package (aka an app) will be developed that enables individuals and groups to: (a) carry out HCS sorting exercises, (b) to explore the knowledge structures generated by those exercises, and (c) to test relationships between attributes of these structures.
Details are available on this password protected web page. Email rick.davies@gmail.com for acccess
EARLIER methods
Variations of hierarchical card sorting
Courtesy of Joachim Harloff, I have come across “Sorting Data: Collection and Analysis“ by A.P.M. Coxon, published by Sage in 1999. Some years later Harloff and Coxon collaborated to produce this useful book How To Sort: A short guide on sorting investigations. There is also this useful 2006 The Method of Sorting website, by Coxon.
On pages 24 to 28 of Coxon (1999) there is a section 2.7.3 on Hierarchy Construction. The first part describes “Agglomerative Hierarchical Sorting” , where the respondent is presented with all N cards as separate 1 member piles. They then asked to reduce the number of piles by 1, by joining the most similar items. Then this task is reiterated, until only one pile remains. The next section then described “Divisive Hierarchical Sorting”, which uses the same initial sorting process as HCS described above, but with some differences discussed below. He credit the first use of divisive sorting to James S Boster, a cognitive anthropologist, in his 1986 journal article ” Can Individuals Recapitulate the Evolutionary Development of Color Lexicons? Ethnology 25(1):61-74. Bolster was looking for lack of difference in color perception across disparate communities as possible evidence of non-cultural determinants of that perception.
The differences between Bolster’s Divisive Hierarchical Sorting” and HCS:
-
- Boster asked people to sort items into two groups based on their similarity, without any further definition of that term. HCS asks people to sort items into two groups according to “what they think is the most significant difference“, where significance is defined in terms of consequences (realised or likely).
- That guidance is based on a specific epistemology developed by Gregary Bateson, who defined information as a difference that makes a difference. Though Boster did have an underling ontological theory, that perceptions of color differences were biologically evolved rather than culturally constructed
- While Boster asked people to describe the nature of the similarity, he made no further inquiries about the sort result. With the HCS follow-up questions are asked. After the difference is described, people are asked for an explanation: “why do you think that difference was most significant”.
- In Boster’s work was no subsequent use of the tree structure to explore preferences, plans and other associated differences between the different categories of items that had been described by the emergent tree structure – as was the case with HCS
extra references
Some extra resources on card/pile sorting and related methods:
-
-
-
- Davies, Rick (2021) Tool Share – Hierarchical Card Sorting (HCS). Video recording of a UK Evaluation Society webinar
- Blanchard, S. J., & Banerji, I. (2016). Evidence-based recommendations for designing free-sorting experiments. Behavior Research Methods, 48(4), 1318–1336.
- Harloff, J., & Coxon, A. P. M. (2007). How To Sort; A short guide on sorting investigations.
- Borgatti, S. P. (Ed.). (1999). Elicitation Techniques for Cultural Domain Analysis. In Designing and Conducting Ethnographic Research (Ethnographer’s Toolkit).
- Coxon, A. P. M. (1999). Sorting data: Collection and analysis. SAGE.
- Gladwin, C. H. (1989). Ethnographic decision tree modeling.
-
-
To do any type of sorting exercise a list of cards to be sorted is necessary. Such lists can be identified/generated using unstructured interviews, or by using more structured ethnographic methods such as freelisting. Some suggested reading:
- Borgatti, S. (1998). Elicitation Techniques for Cultural Domain Analysis. In The Ethnographer’s Toolkit (Vol. 3). Altimira Press.
-
Quinlan, M. (2019). The Freelisting Method (pp. 1431–1446). https://doi.org/10.1007/978-981-10-2779-6_12-2
-
Placek C, Budzielek E, White L, et al. (2023) Anthropology in Evaluation: Free-Listing to Generate Cultural Models. American Journal of Evaluation: 109821402211160. DOI: 10.1177/10982140221116095.
Design by Rick Davies
2 thoughts on “Hierarchical Card Sorting (HCS)”