See also this newer page Exploring the text analytic capacities of GPT4
Introduction to ChatGPT, by ChatGPT
I asked ChatGPT this question: “Describe ChatGPT in 200 words, for a general, non-specialist reader”
The response: “ChatGPT is a language model developed by OpenAI. It is based on a deep learning technique called transformer, which allows it to generate human-like text. It has been trained on a large dataset of text from the internet, and can be fine-tuned for specific tasks such as language translation, text summarization, and text generation. ChatGPT is capable of understanding and generating text in multiple languages, and can respond to a wide range of questions, prompts, and commands. It can also generate creative content such as stories and poetry. The model can be used for various applications like virtual assistants, chatbots, automated writing and more. However, it is important to note that while ChatGPT is highly advanced, it is not a human and may not always understand context or nuances in language and may produce errors or biases present in the training data”.
Here is an introductory video providing a beginners guide to how to access and use ChatGPT: https://www.youtube.com/watch?v=JTxsNm9IdYU
Using Chat GPT to analyses bodies of text
Why: This is my current interest, where I think ChatGPT is already useful in its current form
The challenge: Evaluators often have to deal with large volumes of text data, including
-
- official documents describing policies and programmes,
- records of individual interviews and group discussions.
Manual analysis of this material can be very time consuming. In recent years a number of different software packages have been developed which are useful for different forms of content analysis. These are generally described as text analytics, text mining and Natural Language Processing (NLP) methods. I have experimented with some of these methods, including clustering tools like Topic Modelling, sentiment analysis methods, and noun and key word extraction tools.
From my limited experience to date, ChatGPT seems likely to leave many of these tools behind. Primarily on criteria such as flexibility and usability. I am less certain on criteria such as transparency of process and replicability of results. I need to give these more of my attention
How it works – from a user perspective
Here below is the user interface, seen after you have logged on. You can see prompt I have written in the top of the white section. Then underneath is the ChatGPT response. I then have two options.
- To click on “Regenerate Response” to create an alternative body of text to the one already shown. This can be done multiple times, until new variant responses are no longer generated. It is important to use this option because in your specific context one response may be more suitable than others, and ChatGPT won’t know the details of your context, unless it is described in the prompt
- To create a new prompt, such as “Simplify this down to 200 words, using less technical language”. The dialogic process of writing prompts, reading results, writing prompts and reading results can go on as long as needed. A point to note here is that ChatGPT remembers the whole sequence of discussion, as context for the most current prompt. But you can start a new chat at any point, and when you do so the old one will remain listed in the left side panel. But it will no longer be part of ChatGPT’s current memory, when responding to the current prompt.
There is a similarity between these two functions and March’s (1991) distinction between two complimentary approaches to learning: Exploration and Exploitation. With regeneration being more exploratory and refined prompts being more exploitative.
But bear in mind that ChatGPT is using data that was available up to 2021. It does not (yet) have real time access to data on the internet. When it does, that will be another major step forward. Fasten your seat belts!
.
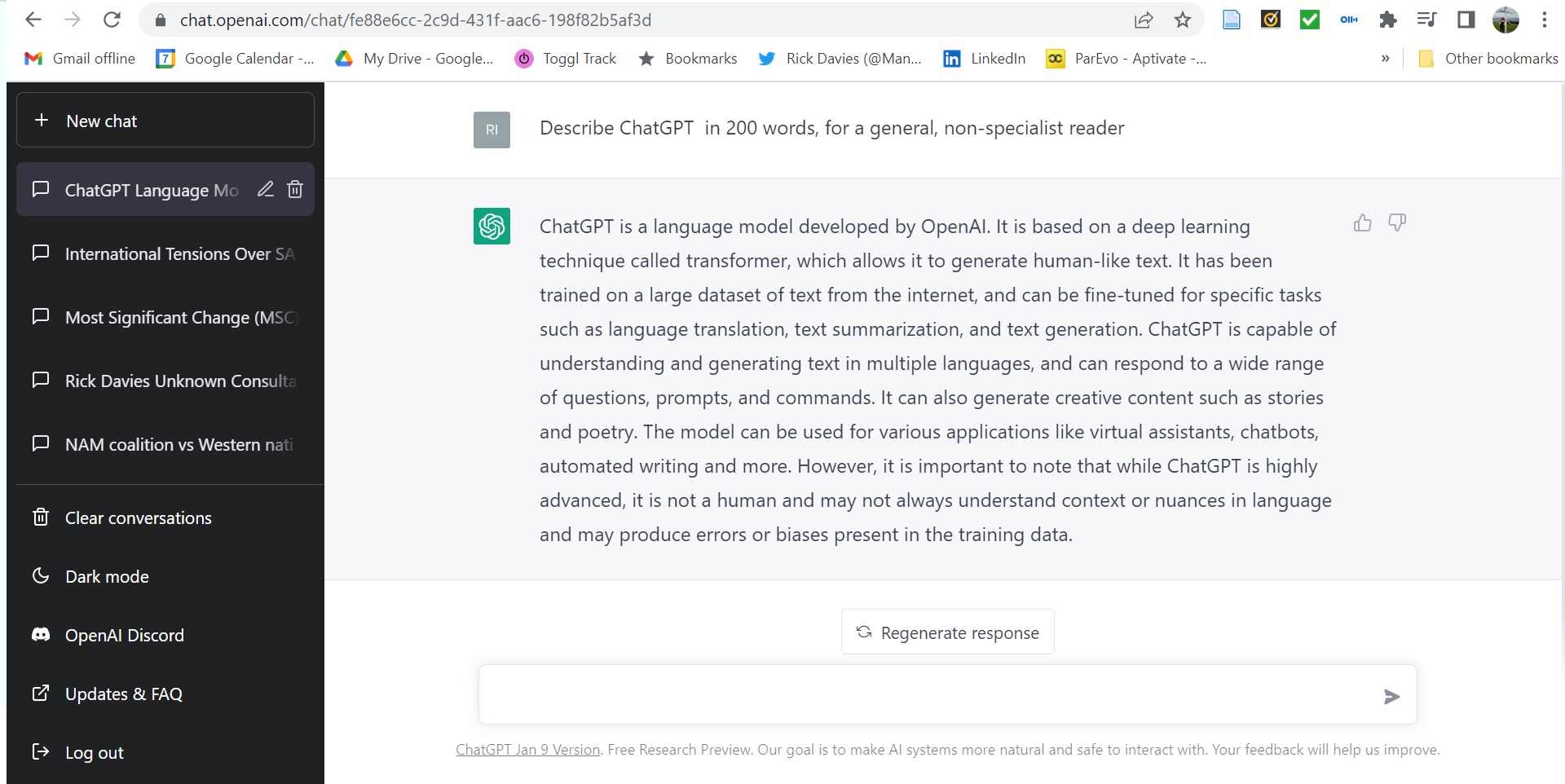
The design of prompts
This is the key to the whole process. Careful design of prompts will deliver more rewards. The more clearly specified your request, the more likely you will see results which are useful.
I will now list some of the prompts, and kinds of prompts, I have experimented with. These have all been applied to paragraphs of text generated by a ParEvo exercise (which I cant quote here for privacy reasons).
-
- Text summarisation
- Summarize the following text in 300 words or less
- Write a newspaper headline for the events described in each of the three paragraphs
- Differentiation of texts
- Identify the most noticeable differences between the events described in the following two paragraphs of text
- Identify three differences between the two paragraphs of text
- Pile sorting
-
- Sort there three paragraphs of text into two piles of paragraphs, and describe what you think is the most significant difference between the two sets of paragraphs, in terms of the events they are describing.
- Evaluation of content on predefined criteria
- All three paragraphs describe imagined futures. Rank these three paragraphs in terms of their optimism, and explain why they have been ranked this way
- All three paragraphs already provided above describe imagined futures. Rank these three paragraphs in terms of their realism i.e how likely the events in the paragraphs could actually happen. Then explain why they have been ranked this way
- Evaluation of content on unspecified criteria
- For each of the three paragraphs provided above, list 5 adjectives that best describe the events in those paragraphs
- Actor extraction
-
- Describe the types of actors mentioned in each of the two piles. By actors I mean people, groups, organisations and states
-
- Using three bullet points, list the people, groups, organisations and countries named in each of these three paragraphs of text.
- Relationship extraction
- Using the list of actors already generated, identify alliances (e.g. commonalities of interests) that exist between any these actor. List these in order of the strength of evidence that that an alliance exists
- Network mapping
- Create an affiliation matrix based on the above data about actors and their relationships, where actors are listed row by row, and the individual alliances are listed by column. The latter can be numbered or named. The cell contents will be 0 or 1, where 1 represents a specific actors membership of a specific alliance and 0 represents non membership
2023 03 03 Postscript. Check out Pickaxe. Here you can design a simple (no code required) app that you and others can use to carry out specific AI based inquiries. Such as sentiment analysis or actor extraction. Try my Pickaxe AI Pile Sorting Exercise Vs 2
Steve Powell, a colleague of mine (who developed the Causal Map app) has been testing the following prompts, with the intention to identify if ChatGPT can usefully identify causal connections between events in a text:
-
- Please look at this sentence and identify the cause and the effect.
- Great, now can you just identify cause and effect pairs in this sentence
- Please do that again but with only the cause and effect pairs you are most sure about
- Thanks, can you do that again so that, when a causal factor (cause or effect) is part of a longer chain, you use the same name for it when you report it as effect in one pair and as cause in another pair?
I have not quoted the original text that was presented for analysis, nor all the results. But here is the response to the last prompt:
-
- Sure, here are the cause and effect pairs in the text that I am most sure about:
Cause: “Organisation 1” Effect: “Changed behaviors in caring for health”
Cause: “Changed behaviors in caring for health” Effect: “Considerably healthy”
Cause: “Prohibitions on going to the hospital” Effect: “Going to church”
Cause: “Use of mosquito nets” Effect: “Decrease in getting sick”
Cause: “Changed behaviors in caring for health” Effect: “Taking three baths per day”
Cause: “Changed behaviors in caring for health” Effect: “Washing hands after visiting the washrooms”
Note that I’ve used the same name “Changed behaviors in caring for health” for the cause in the first and fifth pair and as the effect in the second pair.
Steve also notes that… another important challenge I forgot to mention is to get it to abstract a little, so that it uses the same labels for similar concepts across paragraphs and cases. This is a task that I also felt needs further exploration through better designed prompts, to see if ChatGPT can do concept abstraction or not.
He also reports that he will work a prompt to get it to format its output for more easy upload into Causal Map. That could be very useful for many people, including myself. I have been doing the same kind of prompt development with text descriptions of network relationships to be converted into a data format that can be imported into social network analysis software – see further above.
PS: Read more of Steve’s thoughts and experiment with ChatGPT and causality on his blog here: Causal Map: Making Connections
Other applications that may be useful to evaluators
The one feature that seems to have received the least attention, within the generalist media, is how ChaptGP and its related products Open AI Codex, could have a major impact on software development . “Proficient in more than a dozen programming languages, Codex can now interpret simple commands in natural language and execute them on the user’s behalf” – executing them meaning write relevant functional computer code.
In response a query I made, ChatpGP replied
“As an AI language model, I can help users with coding suggestions and provide information on a wide range of programming languages. Some of the most common programming languages that I can assist with include:
Python: a high-level, interpreted language known for its simplicity and ease of use, often used in data science and machine learning.
Java: a popular object-oriented language used for developing large-scale applications.
JavaScript: a client-side scripting language used for creating interactive web pages and web applications.
C++: a powerful, high-performance language used for building operating systems, games, and other resource-intensive applications.
Ruby: a dynamic, object-oriented language used for web development, especially with the Ruby on Rails framework.
PHP: a server-side scripting language used for building dynamic websites and web applications.
Swift: a modern programming language developed by Apple for iOS, macOS, watchOS, and tvOS development.
C#: an object-oriented language developed by Microsoft, used for developing Windows desktop applications, games, and other applications.
These are just a few examples of the many programming languages I can provide assistance with, and I can also provide guidance on other languages based on the user’s needs and preferences.
What it does not also mention is that it can provide advice on relevant Excel formula, macros and VBA code. A capacity likely to be relevant to a wider group of evaluators
One point to note about this capacity, is that testing the answers is straightforward in most cases. It either works or does not, and if it does work it should be easy enough to identify if the results are correct or not.
There are a few videos available online that explain what can be done by combining use of ChatGPT and Excel:
Quotes of note..
“As the novelty of that surprise wears off, it is becoming clear that ChatGPT is less a magical wish-granting machine than an interpretive sparring partner”
Crypto was money without utility,” he argued, while tools such as ChatGPT are, “for now, utility without money.”
“It’s going to be fascinating to see how people incorporate this second brain into their job,”
“…you’re curious how GPT and other AI tools are going to change “the way people talk about talking, write about writing, and think about thinking.”
“If the old line was “Learn to code,” what if the new line is “Learn to prompt”? Learn how to write the most clever and helpful prompts in such a way that gives you results that are actually useful.”
“Your job won’t be replaced by AI but it may be replaced by someone who knows how to use AI better than you…”
In summary…as of 22 February 2023
Seeing ChatGPT as “…an interpretive sparring partner…” is a good approximate description. Another is that working with ChatGPT is (as others have already said) like working with an intern that has at least a Masters degree (or more) in every subject you need to be working with. The trouble is that this intern is not above bluffing and bullshitting when it cant find any thing better (i.e. more informed/detailed/accurate) to say. So you need to get past the understandable “Wow” reaction to its apparent intelligence and creativity, and lift your own game to the level where you are ready and able to critically review what ChapGPT has responded with. Then, through further dialogue with ChatGPT, get it to know when some of its answers are not acceptable and, through further feedback, to improve on its own performance thereafter.
Which will of course mean you will then (again) need to get past any (additional) “Wow” reaction to its (additional) apparent intelligence and creativity, and lift your own game to (an additional) another level where you are ready and able to critically review what ChapGPT has responded with”…. :-) The ball comes back into your court very quickly. And it does not show evidence of tiring, no matter how long the dialogue continues.
Lame criticisms – a miscellany with some responses
- But the data its responses are based on is biased. Yes, true. Welcome to the world. All of us see the world through a biased sample of the world and what it has to offer. With AI like ChatGP we have an opportunity, not yet realised, to be able to see the nature of that bias…what kind of data has been included and what kind has been excluded.
- But it gets things wrong. Yes, true. Welcome to the world. So do we humans. When this seems to be happening we often then ask questions, and explore different approaches. ChatGPT builds in four options of this kind. As explained above. 1. Ask follow up queries, 2. Regenerate a response, 3. Channel feedback via the thumbs up/down, 4. Start a new chat. The clue is in the name “chat” i.e dialogue, to use a fancier name.
- It is/is not sentient/conscious. I am just not sure if this is a helpful claim or debate. All we have access to is its behavior, not interior states, whatever shape of form they may take, if any. Again, perhaps, welcome to the world, of humans and other beings. We do know that AI, like ChaGPT, can be asked to respond in the style of x type person or entity. As we also are, when we take on different social roles. In future, when its data base is updated to include post November 2022 information, that will include data about itself and how various humans have reacted to and think about ChatGPT. It will have a form of self-knowledge, acquired via others. Like aspects of ourselves. But probably a lot more diverse and contradictory than the social feedback that individual’s generally get. How will that effect its responses to human prompts thereafter, if at all, I have no idea. But it does taken me into the real of values or meta-rules, some of which it must already have, installed by its human designers, in order to prevent presently foreseeable harms. This takes us into the large and growing area of discussion around the alignment problem (Christian, 2020)
PS: There seem to be significant current limitations to ChatGPT’s ability to build up self-knowledge from user responses. Each time a new Chat is started no memory is retained of the contents of previous chats (which include users responses). Even within a current chat there appears to be a limit on how many prior prompts and associated responses (and the information they all contain), can be accessed by ChatGPT.